What Is the Definition of Machine Learning?

The method learns from previous test data that hasn’t been labeled or categorized and will then group the raw data based on commonalities (or lack thereof). Cluster analysis uses unsupervised learning to sort through giant lakes of raw data to group certain data points together. Clustering is a popular tool for data mining, and it is used in everything from genetic research to creating virtual social media communities with like-minded individuals. You can foun additiona information about ai customer service and artificial intelligence and NLP. Machine learning is used in many different applications, from image and speech recognition to natural language processing, recommendation systems, fraud detection, portfolio optimization, automated task, and so on. Machine learning models are also used to power autonomous vehicles, drones, and robots, making them more intelligent and adaptable to changing environments. Natural language processing is a field of machine learning in which machines learn to understand natural language as spoken and written by humans, instead of the data and numbers normally used to program computers.
This type of knowledge is hard to transfer from one person to the next via written or verbal communication. However, not only is this possibility a long way off, but it may also be slowed by the ways in which people limit the use of machine learning technologies. The ability to create situation-sensitive decisions that factor in human emotions, imagination, and social skills is still not on the horizon. Further, as machine learning takes center stage in some day-to-day activities such as driving, people are constantly looking for ways to limit the amount of “freedom” given to machines. It is used as an input, entered into the machine-learning model to generate predictions and to train the system. All types of machine learning depend on a common set of terminology, including machine learning in cybersecurity.
The robot-depicted world of our not-so-distant future relies heavily on our ability to deploy artificial intelligence (AI) successfully. However, transforming machines into thinking devices is not as easy as it may seem. Strong AI can only be achieved with machine learning (ML) to help machines understand as humans do. Still, most organizations either directly or indirectly through https://chat.openai.com/ ML-infused products are embracing machine learning. Companies that have adopted it reported using it to improve existing processes (67%), predict business performance and industry trends (60%) and reduce risk (53%). While this topic garners a lot of public attention, many researchers are not concerned with the idea of AI surpassing human intelligence in the near future.
Machine learning computer programs are constantly fed these models, so the programs can eventually predict outputs based on a new set of inputs. Algorithms then analyze this data, searching for patterns and trends that allow them to make accurate predictions. In this way, machine learning can glean insights from the past to anticipate future happenings. Typically, the larger the data set that a team can feed to machine learning software, the more accurate the predictions. Deep learning is a subfield within machine learning, and it’s gaining traction for its ability to extract features from data.
The robotic dog, which automatically learns the movement of his arms, is an example of Reinforcement learning. Traditional Machine Learning combines data with statistical tools to predict an output that can be used to make actionable insights. Even after the ML model is in production and continuously monitored, the job continues.
Reinforcement learning is a feedback-based learning method, in which a learning agent gets a reward for each right action and gets a penalty for each wrong action. The agent learns automatically with these feedbacks and improves its performance. In reinforcement learning, the agent interacts with the environment and explores it.
A machine learning model is a program that can find patterns or make decisions from a previously unseen dataset. For example, in natural language processing, machine learning models can parse and correctly recognize the intent behind previously unheard sentences or combinations of words. In image recognition, a machine learning model can be taught to recognize objects – such as cars or dogs. A machine learning model can perform such tasks by having it ‘trained’ with a large dataset. During training, the machine learning algorithm is optimized to find certain patterns or outputs from the dataset, depending on the task. The output of this process – often a computer program with specific rules and data structures – is called a machine learning model.
Machine Learning from theory to reality
In a neural network trained to identify whether a picture contains a cat or not, the different nodes would assess the information and arrive at an output that indicates whether a picture features a cat. Decision tree learning uses a decision tree as a predictive model to go from observations about an item (represented in the branches) to conclusions about the item’s target value (represented in the leaves). It is one of the predictive modeling approaches used in statistics, data mining, and machine learning. Tree models where the target variable can take a discrete set of values are called classification trees; in these tree structures, leaves represent class labels, and branches represent conjunctions of features that lead to those class labels. Decision trees where the target variable can take continuous values (typically real numbers) are called regression trees.

As input data is fed into the model, the model adjusts its weights until it has been fitted appropriately. This occurs as part of the cross validation process to ensure that the model avoids overfitting or underfitting. Supervised learning helps organizations solve a variety of real-world problems at scale, such as classifying spam in a separate folder from your inbox. Some methods used in supervised learning include neural networks, naïve bayes, linear regression, logistic regression, random forest, and support vector machine (SVM). Semisupervised learning works by feeding a small amount of labeled training data to an algorithm. From this data, the algorithm learns the dimensions of the data set, which it can then apply to new unlabeled data.
DBSCAN Clustering Algorithm Demystified
It can also compare its output with the correct, intended output to find errors and modify the model accordingly. Rule-based machine learning is a general term for any machine learning method that identifies, learns, or evolves “rules” to store, manipulate or apply knowledge. The defining characteristic of a rule-based machine learning algorithm is the identification and utilization of a set of relational rules that collectively represent the knowledge captured by the system. Reinforcement learning is an area of machine learning concerned with how software agents ought to take actions in an environment so as to maximize some notion of cumulative reward. In reinforcement learning, the environment is typically represented as a Markov decision process (MDP).
They use historical data as input to make predictions, classify information, cluster data points, reduce dimensionality and even help generate new content, as demonstrated by new ML-fueled applications such as ChatGPT, Dall-E 2 and GitHub Copilot. Machine learning (ML) is a type of artificial intelligence (AI) focused on building computer systems that learn from data. The broad range of techniques ML encompasses enables software applications to improve their performance over time. The original goal of the ANN approach was to solve problems in the same way that a human brain would.
However, over time, attention moved to performing specific tasks, leading to deviations from biology. Artificial neural networks have been used on a variety of tasks, including computer vision, speech recognition, machine translation, social network filtering, playing board and video games and medical diagnosis. Most of the dimensionality reduction techniques can be considered as either feature elimination or extraction.

This approach involves providing a computer with training data, which it analyzes to develop a rule for filtering out unnecessary information. The idea is that this data is to a computer what prior experience is to a human being. Machine learning has been a field decades in the making, as scientists and professionals have sought to instill human-based learning methods machine learning définition in technology. Most computer programs rely on code to tell them what to execute or what information to retain (better known as explicit knowledge). This knowledge contains anything that is easily written or recorded, like textbooks, videos or manuals. With machine learning, computers gain tacit knowledge, or the knowledge we gain from personal experience and context.
What are the different machine learning models?
Signals travel from the first layer (the input layer) to the last layer (the output layer), possibly after traversing the layers multiple times. Similarity learning is an area of supervised machine learning closely related to regression and classification, but the goal is to learn from examples using a similarity function that measures how similar or related two objects are. It has applications in ranking, recommendation systems, visual identity tracking, face verification, and speaker verification. A core objective of a learner is to generalize from its experience.[6][43] Generalization in this context is the ability of a learning machine to perform accurately on new, unseen examples/tasks after having experienced a learning data set. Supervised learning involves mathematical models of data that contain both input and output information.
A regression model uses a set of data to predict what will happen in the future. In an underfitting situation, the machine-learning model is not able to find the underlying trend of the input data. When an algorithm examines a set of data and finds patterns, the system is being “trained” and the resulting output is the machine-learning model. Then, in 1952, Arthur Samuel made a program that enabled an IBM computer to improve at checkers as it plays more. Fast forward to 1985 where Terry Sejnowski and Charles Rosenberg created a neural network that could teach itself how to pronounce words properly—20,000 in a single week. In 2016, LipNet, a visual speech recognition AI, was able to read lips in video accurately 93.4% of the time.
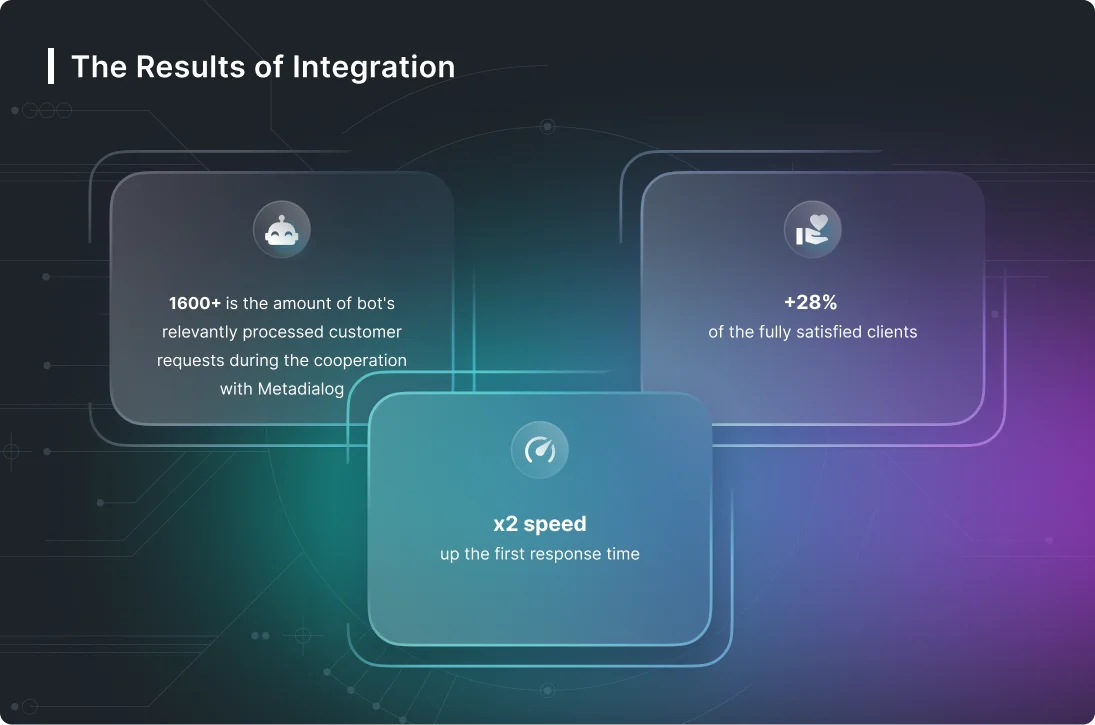
ML finds application in many fields, including natural language processing, computer vision, speech recognition, email filtering, agriculture, and medicine.[4][5] When applied to business problems, it is known under the name predictive analytics. Although not all machine learning is statistically based, computational statistics is an important source of the field’s methods. Semi-supervised learning falls in between unsupervised and supervised learning. For example, when someone asks Siri a question, Siri uses speech recognition to decipher their query. In many cases, you can use words like “sell” and “fell” and Siri can tell the difference, thanks to her speech recognition machine learning. Speech recognition also plays a role in the development of natural language processing (NLP) models, which help computers interact with humans.
Meaning of machine learning in English
This ability to learn from data and adapt to new situations makes machine learning particularly useful for tasks that involve large amounts of data, complex decision-making, and dynamic environments. Some manufacturers have capitalized on this to replace humans with machine learning algorithms. Machine learning algorithms are trained to find relationships and patterns in data.
Machine learning’s use of tacit knowledge has made it a go-to technology for almost every industry from fintech to weather and government. There are a few different types of machine learning, including supervised, unsupervised, semi-supervised, and reinforcement learning. In reinforcement learning, the algorithm is made to train itself using many trial and error experiments. Reinforcement learning happens when the algorithm interacts continually with the environment, rather than relying on training data. One of the most popular examples of reinforcement learning is autonomous driving.
- He applies the term to the algorithms that enable computers to recognize specific objects when analyzing text and images.
- Reinforcement learning is another type of machine learning that can be used to improve recommendation-based systems.
- The system uses labeled data to build a model that understands the datasets and learns about each one.
- Machine learning ethics is becoming a field of study and notably be integrated within machine learning engineering teams.
- He defined machine learning as – a “Field of study that gives computers the capability to learn without being explicitly programmed”.
Some popular examples of machine learning algorithms include linear regression, decision trees, random forest, and XGBoost. Unsupervised machine learning algorithms are used when the information used to train is neither classified nor labeled. Unsupervised learning studies how systems can infer a function to describe a hidden structure from unlabeled data. Several learning algorithms aim at discovering better representations of the inputs provided during training.[62] Classic examples include principal component analysis and cluster analysis. This technique allows reconstruction of the inputs coming from the unknown data-generating distribution, while not being necessarily faithful to configurations that are implausible under that distribution. This replaces manual feature engineering, and allows a machine to both learn the features and use them to perform a specific task.
Machine learning-enabled programs come in various types that explore different options and evaluate different factors. There is a range of machine learning types that vary based on several factors like data size and diversity. Below are a few of the most common types of machine learning under which popular machine learning algorithms can be categorized. Unsupervised learning is a type of machine learning where the algorithm learns to recognize patterns in data without being explicitly trained using labeled examples. The goal of unsupervised learning is to discover the underlying structure or distribution in the data.
Google’s AI algorithm AlphaGo specializes in the complex Chinese board game Go. The algorithm achieves a close victory against the game’s top player Ke Jie in 2017. This win comes a year after AlphaGo defeated grandmaster Lee Se-Dol, taking four out of the five games. The device contains cameras and sensors that allow it to recognize faces, voices and movements.
It can also predict the likelihood of certain errors happening in the finished product. An engineer can then use this information to adjust the settings of the machines on the factory floor to enhance the likelihood the finished product will come out as desired. With error determination, an error function is able to assess how accurate the model is. The error function makes a comparison with known examples and it can thus judge whether the algorithms are coming up with the right patterns. George Boole came up with a kind of algebra in which all values could be reduced to binary values.
Unsupervised Learning
Instead of spending millions of human hours on each trial, machine learning technologies can produce successful drug compounds in weeks or months. The healthcare industry uses machine learning to manage medical information, discover new treatments and even detect and predict disease. Medical professionals, equipped with machine learning computer systems, have the ability to easily view patient medical records without having to dig through files or have chains of communication with other areas of the hospital. Updated medical systems can now pull up pertinent health information on each patient in the blink of an eye. Many people are concerned that machine-learning may do such a good job doing what humans are supposed to that machines will ultimately supplant humans in several job sectors. In some ways, this has already happened although the effect has been relatively limited.
Overall, machine learning has become an essential tool for many businesses and industries, as it enables them to make better use of data, improve their decision-making processes, and deliver more personalized experiences to their customers. Much of the technology behind self-driving cars is based on machine learning, deep learning in particular. In some cases, machine learning can gain insight Chat PG or automate decision-making in cases where humans would not be able to, Madry said. “It may not only be more efficient and less costly to have an algorithm do this, but sometimes humans just literally are not able to do it,” he said. With the growing ubiquity of machine learning, everyone in business is likely to encounter it and will need some working knowledge about this field.
For example, the car industry has robots on assembly lines that use machine learning to properly assemble components. In some cases, these robots perform things that humans can do if given the opportunity. However, the fallibility of human decisions and physical movement makes machine-learning-guided robots a better and safer alternative. In the model optimization process, the model is compared to the points in a dataset.

Machine learning plays a central role in the development of artificial intelligence (AI), deep learning, and neural networks—all of which involve machine learning’s pattern- recognition capabilities. In supervised learning, sample labeled data are provided to the machine learning system for training, and the system then predicts the output based on the training data. Machine learning is important because it allows computers to learn from data and improve their performance on specific tasks without being explicitly programmed.
In this way, the other groups will have been effectively marginalized by the machine-learning algorithm. Chatbots trained on how people converse on Twitter can pick up on offensive and racist language, for example. Machine learning starts with data — numbers, photos, or text, like bank transactions, pictures of people or even bakery items, repair records, time series data from sensors, or sales reports. The data is gathered and prepared to be used as training data, or the information the machine learning model will be trained on. When companies today deploy artificial intelligence programs, they are most likely using machine learning — so much so that the terms are often used interchangeably, and sometimes ambiguously. Machine learning is a subfield of artificial intelligence that gives computers the ability to learn without explicitly being programmed.
What is Explainable Artificial Intelligence (XAI)? – Techopedia
What is Explainable Artificial Intelligence (XAI)?.
Posted: Fri, 03 Nov 2023 07:00:00 GMT [source]
Since deep learning and machine learning tend to be used interchangeably, it’s worth noting the nuances between the two. Machine learning, deep learning, and neural networks are all sub-fields of artificial intelligence. However, neural networks is actually a sub-field of machine learning, and deep learning is a sub-field of neural networks.
Determine what data is necessary to build the model and whether it’s in shape for model ingestion. Questions should include how much data is needed, how the collected data will be split into test and training sets, and if a pre-trained ML model can be used. It requires diligence, experimentation and creativity, as detailed in a seven-step plan on how to build an ML model, a summary of which follows. Reinforcement learning works by programming an algorithm with a distinct goal and a prescribed set of rules for accomplishing that goal. A data scientist will also program the algorithm to seek positive rewards for performing an action that’s beneficial to achieving its ultimate goal and to avoid punishments for performing an action that moves it farther away from its goal.
Machine learning is a pathway to artificial intelligence, which in turn fuels advancements in ML that likewise improve AI and progressively blur the boundaries between machine intelligence and human intellect. Alan Turing jumpstarts the debate around whether computers possess artificial intelligence in what is known today as the Turing Test. The test consists of three terminals — a computer-operated one and two human-operated ones.
Unsupervised machine learning can find patterns or trends that people aren’t explicitly looking for. For example, an unsupervised machine learning program could look through online sales data and identify different types of clients making purchases. Recommendation engines, for example, are used by e-commerce, social media and news organizations to suggest content based on a customer’s past behavior. Machine learning algorithms and machine vision are a critical component of self-driving cars, helping them navigate the roads safely. In healthcare, machine learning is used to diagnose and suggest treatment plans. Other common ML use cases include fraud detection, spam filtering, malware threat detection, predictive maintenance and business process automation.